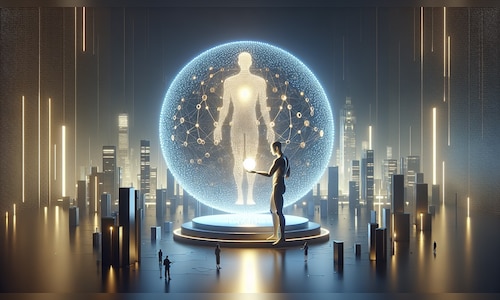
As Generative AI (gen AI) becomes more prevalent, the question that arises is — How safe are these applications?
But what makes it challenging to figure out an answer to the question is large language models (LLMs) exhibiting emergent behaviours, and their unpredictability. Adding to the complexity is the regulations like the EU AI Act which has recently come into effect, imposing penalties as steep as €35 million or 7% of a company’s global annual revenue for non-compliance.
Companies employing general-purpose AI, particularly LLMs, will face heightened scrutiny. Across the globe, regulations demand that enterprises demonstrate responsible data practices, ensure transparency in LLM use, and potentially explain how their models produce specific outputs.
Despite evolving regulations, a joint study by Genpact and HFS Research shows enterprises are still cautious with their own data due to governance concerns, highlighting the need for a strong, responsible AI framework.
Ignoring responsible AI practices can have costly repercussions, including reputational damage and legal exposure. The urgency is prompting enterprises to embed responsible AI initiatives within their broader strategies and allocate the necessary budgets to support them.
Therefore, to address these challenges, we must understand evolving benchmarks first. Companies must look at potential external advancements and ecosystem-wide progress when evaluating risks. We must keep in mind that while traditional static dataset-based evaluation has limitations, they remain valuable tools to gauge preparedness. Responsible AI principals offer guidance by supplementing static evaluations with additional methods for a more holistic view of model capabilities and risks.
Even leading model developers like OpenAI are taking steps towards responsible AI by creating tools such as System Cards, which offer comprehensive insights into a model’s development, safety protocols, and evaluation processes. This approach aligns with new regulatory frameworks, showing how the AI ecosystem is striving to advance capabilities while keeping safety and ethics at the forefront. Following are four key principles for building a stronger, responsible Gen AI strategy:
- Enhance Data Transparency and Accessibility: Gen AI’s reliance on diverse, heterogeneous data introduces risks of bias and ethical concerns, especially if the data is not carefully curated or vetted for fairness and accuracy. Implementing auditing mechanisms, including human oversight, can mitigate these risks, ensuring the model’s output is unbiased and reliable.
- Establish a Centre of Excellence: Pretrained LLMs provide easy access to AI tools but also introduce challenges for businesses, developers, and regulators. AI governance must extend beyond IT professionals to include key stakeholders with a range of expertise — technical, industry-specific, and ethical best practices. Additionally, cross-departmental collaboration is vital in building frameworks that centre on human values and ethics, ensuring that AI-driven decisions reflect broader corporate responsibilities.
- Invest in Upskilling and Workforce Training: Gen AI is known to produce hallucinations – a mix of factual and fictional content, as per studies. Training employees to understand the workings and limitations of AI models is essential for making informed decisions about the reliability of AI-generated information. Establishing clear guidelines for selecting and fine-tuning models can help organisations ensure consistency and accuracy in their outputs. This proactive approach helps teams navigate the inherent uncertainty of LLMs and create outputs that align with organisational goals.
- Strengthen Governance Practices: A unified data and AI governance framework is critical. Companies must establish clear ownership, access controls, and auditing mechanisms for all data and AI assets. Choosing the right governance model— whether centralised or distributed— depends on the organisation’s specific needs, but having a system in place is imperative.
Therefore, security is an integral component of data governance, and extensive testing during development, including red-teaming exercises to identify risks and devise mitigations, has become a standard practice. Responsible AI frameworks guide governance teams in defining risk frameworks and coordinating red-team assessments.
These evaluations are pivotal in early-risk identification and help organisations map out interactions among various personas throughout the gen AI lifecycle, tailored to their unique capabilities.
Weaving Responsible AI into the Organisational Fabric
Adapting to existing governance structures is crucial to managing risk in Gen AI adoption. Instead of creating new committees or approval boards, companies can extend the scope of their current risk frameworks. This approach ensures minimal disruption to decision-making processes while preserving accountability.
Effective risk management relies on robust governance mechanisms. Cross-functional, responsible AI working groups that include business and technology leaders, alongside experts in data privacy, legal, and compliance, are key. To help integrate responsible AI throughout the organisation, here are some best practices to follow:
- Raise Awareness: Develop a comprehensive strategy for communicating responsible AI practices throughout the organisation. Continuous awareness initiatives help embed these principles into the company’s culture, ensuring long-term adherence.
- Create a Plan: As gen AI becomes more accessible, successful implementation hinges on preparation. Start by identifying the most promising use cases and collaborate with the centre of excellence to address potential risks early.
- Be Transparent: Transparency is critical, and companies should openly acknowledge the capabilities and limitations of gen AI, using lessons learned to educate both internal and external stakeholders. By addressing challenges, such as ambiguous problem definitions and overly specific tests, businesses can build clearer assessments of AI performance.
- Build Trust: Enhancing stakeholder confidence requires transparent gen AI tools. Companies should provide resources explaining the decision-making process, incorporate confidence scores to gauge output reliability, and integrate a human-in-the-loop approach to refine model accuracy over time.
The Road Ahead
The market is transitioning from traditional chatbots to LLM-powered agents, which brings more emergent behaviour and unpredictability. This is why establishing responsible AI policies for widespread adoption remains crucial. As enterprises race to integrate gen AI, they face the challenge of navigating a complex governance landscape while ensuring responsible development.
Enterprise customers are increasingly focused on the ethical implications of AI, which adds another layer of responsibility. AI-first companies are now obligated to conduct impact assessments, which evaluate the consequences of AI deployment. This highlights the importance of a robust, responsible AI framework, which helps businesses avoid pitfalls and protect their reputations while maintaining a competitive edge.
Scaling an ethical enterprise requires careful planning — there are no shortcuts. Responsible AI preparedness will serve as a key differentiator for enterprises as they continue their journey toward successful AI adoption.
— Sreekanth Menon is the Global Head of AI at Genpact.